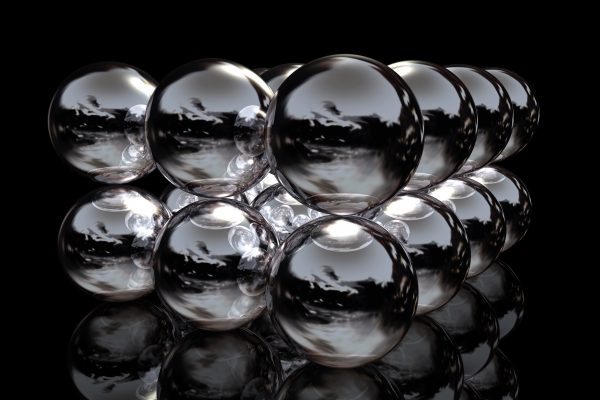
Oct. 03, 2022
Data Quality: Features, Challenges and Best Practices
By Roei Soudai @BlackSwan Technologies
Gartner estimates that poor data quality costs organisations $12.9 million annually on average, and in the long term, it increases the complexity of data ecosystems while reducing the standard of decision-making.
Despite this, a study published by KPMG shows that fewer than 10 per cent of companies have a world-class data strategy for data quality and governance, and this is subsequently costing them a fortune.
It has long been known that an organisation’s data and analytics strategy is only as good as the quality of the data being used. Data quality impacts the performance of analytics tools and ultimately determines the quality of the insights generated, which in turn influences decision-making and key business metrics such as revenue growth and customer satisfaction.
But how do organisations ensure that their data quality is of the required standard? What do they have to take into account? In the Q&A below, Roei Soudai, VP of Products at BlackSwan Technologies, discusses the key elements of data quality, the barriers that organisations are facing, and the strategies that can help organisations achieve higher levels of data quality.
What are the most important aspects of data quality and accuracy?
Data quality is crucial to assess whether the data can serve its purpose. There are a few aspects that can be taken into account, namely reliability, credibility, relevance, and timeliness.
Reliability involves identifying the data relevant to an entity (e.g., a person or a city) based on the entity’s contextual information. Contextualisation excludes irrelevant data from consideration and has the potential to refine data from several aspects. Irrelevant data may derive from unreliable news articles and sources, and websites that have no credible information about entities or outdated information.
There are a number of questions that we can ask ourselves about how best to identify a reliable source. For example, sources from a government or university website are usually reliable, while company websites usually have up-to-date information about a company which is often promotional. Meanwhile, facts on news websites need to be verified to ensure that the information cited can be tracked to the original source.
Credibility means if we believe a source gives us reliable and accurate information. Although these terms sound the same, there is a difference. If a piece of information is reliable, then it is also credible. However, the information’s credibility does not always guarantee its reliability.
Sometimes we can consider dividing sources into primary and secondary sources, where primary sources are usually the original research such as data from surveys, questionnaires, interviews, or observations delivered as pieces of evidence.
Secondary sources, on the other hand, quote other primary sources or other secondary sources. For example, magazine or newspaper accounts of interviews, surveys, or questionnaires conducted by other researchers.
Relevance refers to whether the data is relevant to our request. The source and data can be reliable and credible but the data may not be relevant to what we request to know. Some companies provide strong information without any relevance but it has a cost. Relevance can be solved using AI-based techniques.
Timeliness also relates to relevance, since old data may not be reliable or relevant anymore. Therefore, newer information has a higher impact on the insights we wish to gain from the data.
How should businesses define data quality with respect to their business goals?
There should be an understanding that the quality of data at disposal is directly linked to the impact of a strategic business objective. Organisations use data to extract information to get better insights and make the right decisions. If the data quality is not complete or is substandard it can cause a company to increase its risk exposure, make wrong decisions and ultimately cost the company more financially. Using the data quality factors such as accuracy, completeness, consistency, timeliness, reliability, relevance and credibility can influence the organisation’s business goals.
What are the biggest challenges with ensuring data quality and accuracy? What is the best practice for addressing them?
Today, the biggest challenges in data preparation involve the following aspects:
Data accessibility – not all the information can be accessed due to security aspects on the web, the ability to access special sources and data may involve special techniques for accessing and scrapping the relevant data. Organisations require the capability to fetch data from any type of source.
Data cleansing – in many occasions the data involves a lot of “noise”, for example, advertisements, blog engines, and comments that need to be cleaned during data retrieval.
Data centralisation and standardisation – Most of the barriers preventing businesses from achieving their data quality and accuracy goals result from relying on a traditional, centralised approach to data. As organisations grow, the number of operational sources that it relies on grows, and this creates data silos. Enterprises attempt to fix this by consolidating the data from these sources into one central repository, but this leads to issues with data quality.
How? Firstly, businesses need to unify the data formats of all data sources. This is a time-consuming task that is difficult to get right, so some data is left unformatted or consumed when outdated. As there are many different operational systems, data becomes unsynchronised with some information accurate and some out-of-date. Meanwhile, product owners in different departments who create and consume the data are separated from the data engineers who work on the data, resulting in data discrepancies. This centralised approach makes it more difficult to integrate data into the organisation’s schema.
A decentralised approach to data using a design concept that Gartner and Forrester call the Data Fabric – can help enterprises. It allows enterprises to achieve a single point of visibility into the data across their organisation through data virtualisation, which enables data assets to be directly accessed at any operational source.
Although data silos still exist, they are bypassed; and data sources and providers are consolidated to ensure that departments have access to up-to-date information at any time. With data assets more accessible, organisations can harmonise data sharing between teams and minimise discrepancies between data consumers and engineers. The Data Fabric also allows organisations to standardise data from any source into any schema format.
Overall, this decentralised approach means it won’t take as much time, effort or investment to integrate new data sources. In addition, the intuitive view of virtualised datasets would ensure that the data is up-to-date. This will enable greater enforcement over data consumption and data monetisation capabilities.
How does BlackSwan help organisations address their data quality challenges?
We help organisations to improve their data quality challenges by providing them with our very own Data Fabric implementation within our ELEMENT™ platform. This facilitates data virtualisation and enables data integration and management through AI-powered tools.
Organisations can use our Data Fabric to integrate entity data from all of their internal sources. It constructs a unified, virtual view of all the data within an organisation by cataloguing the metadata of all internal data assets. This allows departments to easily access data from where it originally resides. Our Data Fabric can also integrate data from external sources like open source intelligence and paid sources to further enrich the indexed data.
Our entity resolution methods ensure that all data catalogued are free from noise. Embedded knowledge graphs relate all the data available about each entity, while machine learning algorithms accurately distinguish between entities with similar identifying information. This ensures that the most precise descriptive values are always associated with each entity.
Roei Soudai is Vice President of Products at BlackSwan Technologies. He has close to 20 years industry experience in enterprise software product development and management.
Find out more about ELEMENT™ here.
Follow BlackSwan on Twitter and LinkedIn