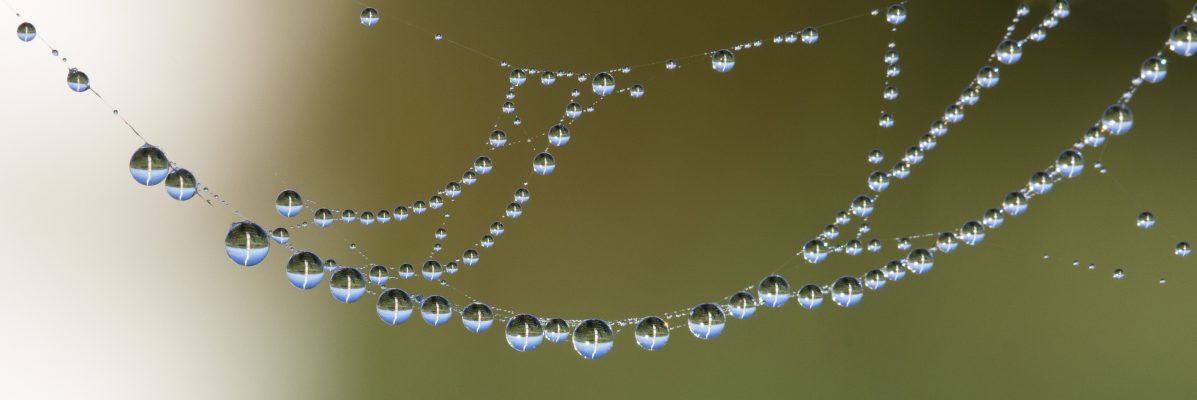
Jun. 16, 2020
Knowledge Graph part 2 – Unleash the power of your data.
By Szymon Klarman @BlackSwan Technologies
Is there more value in the use of a knowledge graph than just a way to uncover non-obvious and indirect relationships hidden in your data, to make better business decisions, as shown in the first part of this series?
Let us look at three real applications and observe how liberating data stored in different information silos becomes the urgent need of today’s enterprises and organisations.
“The continued survival of any business will depend upon an agile, data-centric
architecture that responds to the constant rate of change.”
Donald Feinberg, vice president and distinguished analyst at Gartner
Modern enterprises are extremely data-rich. Furthermore, the Web, as an information publishing and exchange platform, offers vast amounts of data as a commodity.
All this data, however, is spread across multiple disconnected data silos making an efficient collection, aggregation, and reconciliation expensive and time-consuming. According to a survey conducted by Data Economy magazine, 57% of 500 respondents agreed that their organizations struggle with data silos.
“An information silo, or a group of such silos,
is an insular management system
in which one information system or subsystem
is incapable of reciprocal operation with others
that are, or should be, related.”
Wikipedia
The critical value offer of knowledge graphs lies in breaking that data silos and reducing the price of gaining accurate insights into all relevant information in its full context – making it actionable.
Examples of novel applications within data-intensive organisations, especially suited for the use of knowledge graphs, include genomics research and disease understanding, risk mitigation regarding oil wells and drilling, evaluation of legal contracts, or reviewing material hazard datasheets. In this blog, we will be looking at examples of practical applications in pharma, virtual assistants, online shopping, and company research.
We will advocate the power of a knowledge graph, not as another, better silo, with a copy-paste of data to a different database, but a source of continuous, consistent, accessible and up-to-date view of all the relevant knowledge in a single connected graph.
Pharma
Research to create a new drug is highly focused on the analysis of connections – between a disease or condition, symptoms, drug effects, testing conditions, and population. The drug research-related information often comes from multiple sources (silos) and has various formats. The data may come from internal (the pharmaceutical company conducting the research) and external (government agencies, public data, other companies, universities, regional research teams) sources. Further yet, information about related chemical compounds is stored in several chemical libraries and data banks. The overall volume of all relevant data is so high, that manual normalization and processing of it cannot be done effectively.
In this instance, knowledge graph technology allows for automated aggregation of this data into a common source of truth, revealing patterns, correlations, gaps, and anomalies. Further application of AI technology facilitates testing out hypotheses and creating predictions. With this approach, subject matter experts can focus only on the information that is useful and relevant for a current task (noise removed) – to make the research processes faster and more cost-efficient.
The cost of developing a new drug is in the range of Billions US dollars, with a greater than 90 percent failure rate associated with the discovery process. The cost also seems to be rising over time. Shaving off a percentage of this cost by ensuring a single source of truth, data accessibility and allowing the researcher to focus on the most relevant area is a tremendous help.
Virtual assistants and Online Shopping
To provide meaningful conversation, Siri, Alexa, and Google Home are all powered by proprietary knowledge graphs – integrated collections of information about entities existing in the world and links between them – the source of truth about the real world. So, when you ask one of these virtual assistants a question about common topics, you get a meaningful answer. The relevance of this answer depends on the number of contextual cues the assistant can relate. And again, this data is under constant change and comes from numerous sources and in different formats.
The more comprehensive and up to date the knowledge of the assistant is, the more informative and realistic the conversation is. This, in turn, contributes to the usefulness of the service, user experience, and finally, the market share.
Similarly, customer service shopping bots are increasingly built on top of knowledge graphs, which help relate the customer query to the existing inventory. The knowledge graph encapsulates shopping behaviour patterns to bridge the gap between the structured query and behaviour data, to lead the conversation with the best follow-up questions and to present the best results in the least amount of time. Again, relevant and up to date knowledge, allows the assistant to precisely follow the needs and likes of the customers over changes in fashion, seasons, and regional requirements. The better the understanding, the more products are sold to returning customers.
Company Research
Another use-case is company research, which involves an analysis of numerous entities, including organisations, people, corporate deals, jurisdictions, and other, with their rich networks of associations via ownership, shareholding, deal involvement, and other relationships.
To conduct company research, an analyst has to access and review tens of internal databases, knowledge platforms, documents, spreadsheets, and then crawl some public APIs, websites and news sources, just to obtain reasonably comprehensive coverage of the legal entities in question, before the proper analysis can even start. This process is time-consuming, error-prone, and not scalable, thus significantly increasing the cost of making informed decisions.
Once the single source of truth in the form of a knowledge graph is set up, it is easy to answer questions like Who are the direct and indirect subsidiaries of a company? Who is the ultimate parent of a company? Is any of the (in)direct shareholders registered in a specific country? Further, advanced network analytics helps to get better insights into the structure of the domain as a whole, answering questions such as: Who is the most important shareholder in the network?
This approach makes the processed data model highly available and is also flexible and extensible. The underlying graph data model can be used in a data-first or schema-late fashion, which means that any structural constraints applied to the data can be easily deferred or relaxed whenever needed. This offers potential in data integration scenarios for highly evolving and dynamic environments, where entities and relationships of new types can be added without much additional engineering.
As a result, the knowledge graph becomes a base for a current, 360-degree view of any entity. If combined with automated workflow and AI technologies for processing and evaluation, it can lead to tremendous business gains. In applications where this approach was employed in the compliance domain in large financial organisations, the following happened:
– eliminating false positives in company research allowed to decrease operational compliance costs by 50%
– analyst productivity was increased by 100%
– the cost of onboarding a business customer was reduced to about $150. (read more)
With these examples at hand, let us have a more detailed look at the knowledge graph technology and better understand how it can be used as a building block of a larger system.
How many knowledge graphs do you need? Reusing data in a novel context.
As shown above, knowledge graphs become increasingly used as the backbone of sophisticated AI systems that often need to cater for a wide range of applications and downstream analytical processes. As the data model is focused directly on real-world things, it is much easier to repurpose the available data by creating new views of desirable subsets, even in cases which were not foreseen upfront. Once knowledge graphs are created, companies can find a way to exploit them through new business models.
The quality and adequacy of a knowledge graph for a given application can be evaluated against some common criteria, such as: coverage (inclusion of all relevant data), correctness (is all the factual data in the graph correct), freshness (is the included information up to date). If additional information is needed for a new problem to solve, it is usually easy to add another data source and feed the new data into the existing graph. For example, a pharmaceutical company may decide to use the knowledge graph about pharmaceuticals, their effects, suppliers, patent applications and localization to do competitive research, and find a novel offering for an underserved market segment, based on regional need and price point. Online shopping companies can leverage data on customer behaviour to adjust the product range, local offering, and promotions.
How easy is it to create a knowledge graph?
Building a knowledge graph does not come as free lunch and involves some heavy lifting for data science and engineering, whose aim is to automate and scale to the maximum possible extent the steps taken by human analysts:
- recognizing mentions of all named as well as previously unknown yet relevant entities described in different sources
- identifying facts, such as attributes of the entities and relationships holding between them
- reconciling all this information, removing potential ambiguities, duplications, and inconsistencies
- assessing its correctness relative to the trust in the specific sources, ensure it is up to date and contextually scoped
- aligning it under a single semantic data model that is correctly interpretable both by humans as well as the software agents that support its management and analysis
As the sources can vary from structured databases to semi-structured data documents and API responses, through websites, to text documents, the techniques and tools required to conduct this process cover a wide range of modern and more traditional AI, data science and data management methods.
In general, the full life-cycle of knowledge graph creation and maintenance is:
- Continuous – it is not about creating another data silo – a one-off copy-paste of data to a different database. It is about maintaining a consistent, accessible, and up-to-date view of all the relevant knowledge in a single connected graph.
- Iterative – progressing bottom-up, from sources, to metadata cataloging, to semantic integration and reconciliation, to insights. The feedback from each stage is critical to improving the process at the lower levels.
- Socio-technological as it requires the participation of numerous stakeholders: data owners, engineers, analysts, etc. It also encourages and is contingent on a shift in an organisation’s culture to knowledge-centric one.
Single, flexible source of truth available for your organisation.
By incorporating data from various sources and providing a single source of truth, the knowledge graph becomes one of the building blocks for a growing number of AI and process automation applications, allowing to enhance the user experience, reduce cost, and increase efficiency.
An enterprise knowledge graph is at the heart of BlackSwan Technologies’ flagship offering, ELEMENT(™), which is a SaaS\PaaS Enterprise AI-Operating System. This system empowers data-intensive organisations to accelerate business transformation, improve bottom-line performance and top-line revenues, by building enterprise-grade robust AI applications.
ELEMENT(™) collects internal, external, structured and unstructured, in motion or at rest data from any source and constructs a knowledge graph, which is the core element of the Contextual Analytics functionality of the system. When Contextual Analytics is combined with other pillars of the system: Big Data, Artificial Intelligence, and Cognitive Computing, the input data is transformed into insights, then knowledge and wisdom – to estimate risk, predict outcomes or present valuable suggestions.
“By 2023, graph technologies will facilitate rapid contextualization for decision making
in 30% of organizations worldwide.”
Gartner
Read more about the capabilities of ELEMENT(™) in the third part of this series.