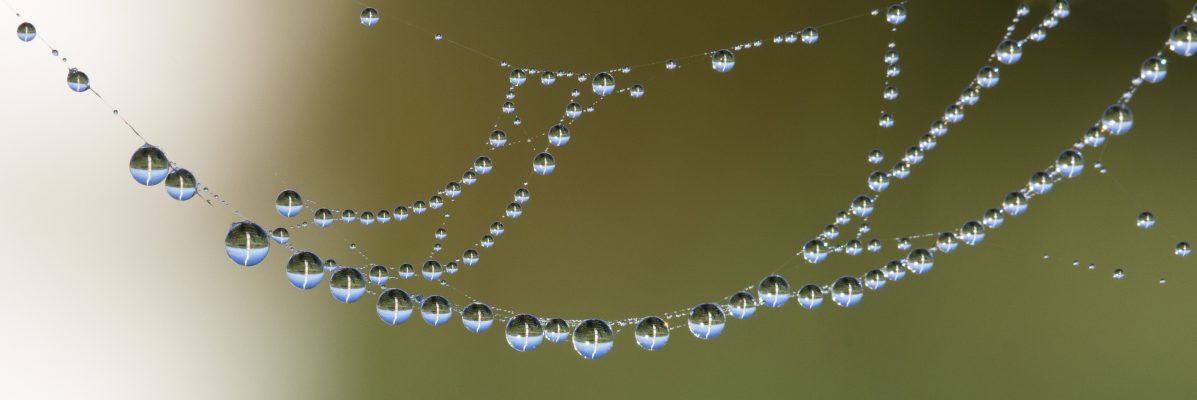
Sep. 23, 2020
Knowledge Graph part 3 – The power at your fingertips.
By Szymon Klarman & Adam Krolak @BlackSwan Technologies
ELEMENT™ is an AI operating system designed by BlackSwan Technologies. The incorporation of the latest knowledge graph (KG) science – as explained in the previous parts of this series – brings unprecedented advantage at several steps needed to develop new ideas into deployable, AI-powered, enterprise-grade applications.
The use of the knowledge graph technology allows achieving greater flexibility and better insights, by employing advanced graph algorithms for data analysis. It also saves computation power and storage resources. To explain the benefits of this approach in ELEMENT™, this article will review the typical steps in developing an AI-powered business application.
Data gathering into a single source of truth
Data-intensive organisations often use a variety of internal data storage systems and external data sources. To use AI reasoning, all relevant information needs to be consolidated as a single source of truth and made accessible for analysis. A traditional process of data consolidation is lengthy and bound for errors. When using KG technology, there is no need to replicate existing data from many different databases into one more, extensive storage database or “data lake”. Instead, a “live” view of all relevant information is maintained in the form of an up-to-date knowledge graph.
Entity driven approach is a second factor significantly boosting the functionality of ELEMENT™. The knowledge graph is a base for identifying entities (a person, a product, an idea, etc.), and the relationships between them, all described with the massive amount of data in different repositories and systems. ELEMENT™ is using proprietary natural language processing (NLP), document ingestion methods, and semantic technology. This allows extracting all meaningful information from available structured and unstructured data spread across various data silos, internal or external to the enterprise. ELEMENT™ “Bring Your Own Schema” approach, means that the features (descriptions) of the relevant data structures can be freely configured by the user, to match any business case and domain knowledge model. The descriptions can later be easily modified where a subset of the same data needs to be used for a different business case (application). This entity- and relationship- based approach enables boundless flexibility to add new features (descriptions) to existing entities. It also makes it very efficient to update the information when it changes or to add completely new entities as needed, thus making the data always up to date and relevant.
When creating KG, ELEMENT™ offers prebuilt and easy to use tools to compare and resolve differences between entities or to complete missing information using state of the art enrichment functionality based on advanced search in internal and external sources.
Data analysis and visualization
In the following step of building an AI application, once the single source of truth is ready, ELEMENT™ provides one of the more exciting features enabled by KG – the link analysis. Link analysis is a data analysis technique to investigate networks and relationships between entities (organisations, people, transactions, etc.). For example, the powerful network analysis capabilities offered by ELEMENT™ are widely used for the investigation of criminal activities such as fraud detection, counter-terrorism, or computer security evaluation. Link analysis is often used for market intelligence, customer segmentation, and financial risk evaluation.
On top of the powerful algorithms, ELEMENT offers a robust Visual Link Analysis (VLA) – an interactive user interface providing visual exploration of entities and their connections (Graph 1).
Graph 1: Interactive Visual Link Analysis interface of ELEMENT™. Easy to explore visualisation of entities and relationships.
The intuitive interface enables the analyst to interact with the data and obtain a variety of views to understand different aspects of the data. For example, the VLA Maps view illustrates the relationship between data points and geographical locations (Graph 2). The VLA Timeline view illustrates the changes in the relationships between entities in a selected period.
Graph 2: Interactive Visual Link Analysis interface of ELEMENT™. Example of VLA Maps view.
Graph analysis algorithms and the Visual Link Analysis offer unsurpassed functionality for fast and intuitive segmentation, risk and opportunity discovery, as well as an easy exploration of domain key players, dependencies, etc.
ELEMENT™ comes with a complete set of building blocks to further analyse the gathered and consolidated data using AI technologies for identification, clustering, trend analysis, hypothesis evaluation, and predictions.
Artificial Intelligence techniques can be easily combined with embedded statistical analysis and deep business rules for advanced processing and decision making. The use of high-performance graph ML algorithms helps analyse all data in various contexts, looking far beyond single dimension analysis of data points.
Business application framework and deployment
Correctly working Machine Learning model is not the end goal of a business application. The model still needs to be deployed to production and often requires a user interface dashboard. On top of this, the completion of specific business tasks requires business workflows.
Knowledge Graph as a temporal source of truth and powerful graph analysis algorithms together with the results of AI insight, change the way analysts approach solving business problems and the need for extended business workflows. ELEMENT™ changes the approach from pull to push. A pull approach means that the analyst has to collect and evaluate all data, solve inconsistencies and search for answers. The push approach means that ELEMENT™ does the “heavy lifting” by performing the labour-intensive tasks and providing the analyst with a processed business problem, reasoning, explanations, and relevant data for decisioning. The analyst can explore and question the data intuitively and efficiently by focusing only on the expertise related tasks.
ELEMENT™ users can leverage the built-in visual workflow creation interface based on the BPMN 2.0 standard. Creation of customised user dashboards to interact with the processed data, collaborate within a business organisation, and complete the business workflow is fast and does not require manual programming.
Additionally, as an end to end AI operating system, ELEMENT™ comes with an underlying framework for the scalable, serverless deployment of the application, while ensuring military-grade access security and data governance.
Sample use cases
ELEMENT™ AI operating system has been implemented in many industries, to speed up digital transformation, lower operating cost, and enable new insight into business risks and opportunities.
For example, the advanced KG-based network analysis is applied to the evaluation of the insurance-related risk. When an insurance company receives a new request to cover a large cargo shipment, ELEMENT™ recognises the owner of the shipment as a relative of a suspected drug trafficker mentioned by a sanctions watchlist. The recognition is precise and automatic, even where there is no direct similarity of address or family name.
Another example relates to customer segmentation and prioritization. A large company uses AI learning to understand what is the real customer value for products and services offered by different departments of the company. ELEMENT™ uses data from several structured and unstructured sources (customer transactions, opinions, relationship history, etc.) to build a complete view of the customer and understand the origin of the customer’s perceived value for each product. This is a base for precise segmentation, tailored offering, and effective initiatives for growth of customer base.
In both of the above scenarios, ELEMENT™ seamlessly integrates the advanced analytic capabilities with state of the art policy and workflow management to deliver ready to use, scalable, enterprise-grade, cognitive applications. These bespoke applications integrate multiple internal and external sources of information, cognitive computing, and user-facing dashboards, both for data scientists and business users (analysts, decision-makers). While the above stakeholders interact with the data and collaborate to accomplish business goals – the system provides an audit trail, case tracking, and escalations.
ELEMENT™ system comes with enterprise-grade scalability and user management, which allows deploying an application for a global organisation in record time, counted in weeks, and to realize unprecedented ROI coming from monetization of data, improved customer journey and cost-effective business operation.
Learn more about BlackSwan Technologies at www.blackswantechnologies.ai or follow us on Twitter and LinkedIn
Szymon Klarman is a Knowledge Architect at BlackSwan Technologies. He holds a PhD in knowledge representation and reasoning and has over 10 years of experience working in the field as an R&D specialist, consultant, and an academic researcher.