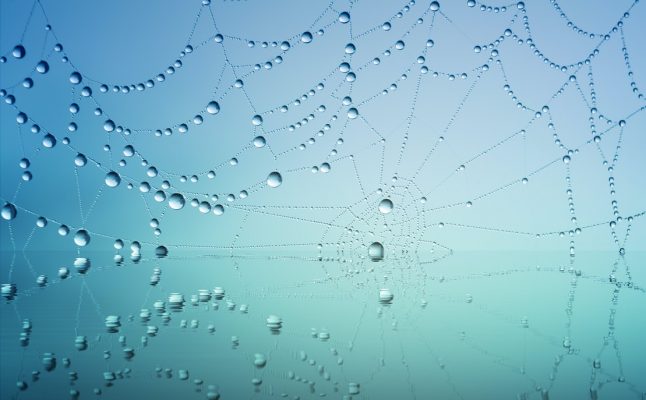
Apr. 12, 2022
Top 5 Capabilities of Effective Knowledge Graphs
By Nadee Wije @BlackSwan Technologies
BlackSwan Technologies recently won the Most Innovative Implementation of Knowledge Graphs Award, here are the capabilities that enabled us to get there.
Knowledge graphs are commonly used by enterprises to contextualise large amounts of structured and unstructured data in a way similar to the human mind. The graphs present entities such as organisations, people, locations, and transactions; and all the relationships between them in a conceptual map, creating a single source of truth.
Knowledge Graphs combine human understanding of real world interdependencies with the power of computation to detect cross-correlations that humans cannot. The graphs enable enterprises to create context and transparency around business decisions, while assessing the far-reaching impacts for any area of the company.
While all knowledge graphs are capable of contextualising data, they are not all the same. Some graphs are more effective than others, especially when combined with other advanced technologies. Here are five game-changing capabilities that can make the difference:
- Schemaless
A schemaless design means that the graph can self-sufficiently fit to any model, entity, relationship or context, helping companies to build any application with ease. The dynamic design allows for flexibility, rapid development iterations and seamless evolution over time as requirements change. Furthermore, it enables firms to repurpose the asset over and over for multiple use cases.
- Composite AI
Knowledge graph capabilities can be enhanced with Composite AI – a combination of AI technologies including natural language processing (NLP), machine learning, and deep learning, which help enrich the graph with relevant entity information and generate insights.
The technologies can enable knowledge graphs to parse and categorise enormous amounts of structured and unstructured data, extracting the relevant entities and context in seconds.
Multi-level semantic NLP, contextual analytics, and data extraction techniques break each unstructured text – such as a news article – down to the sentence level, interpreting an entity’s role in a situation, as well as the nature and severity of the issue, and the involvement of multiple entities.
- Insight Engine
Once you have the context necessary, businesses want to be able to facilitate knowledge discovery – this is where an Insight Engine comes in. An Insight Engine can further analyse the indexed data using visual link analysis (VLA) and graph analysis in conjunction with statistical methods and business rules.
VLA allows analysts to visually explore the connections between entities and obtain a variety of data views such as an illustration of the relationship between entities and geographical locations, or an illustration of the changes in entity relationships over time. This supplements business activities such as market research and customer segmentation; as well as investigations into financial crimes such as fraud, terrorist financing, and money laundering.
Graph analysis on the other hand, in combination with advanced statistical methods and deep business rules, allows analysts to examine data in different contexts for expert-level decision making and to discover risks and opportunities.
- Continuous updates
To remain up-to-date, it’s important that knowledge graphs can continuously scan internal and external repositories of data, open source intelligence, and thousands of public sources such as industry news to build an up-to-date, holistic representation of all relevant entities and the relationships between these entities.
As new information becomes available about the entities indexed on our knowledge graph, such as business partnership announcements, the graph can evolve to reflect the most up-to-date state of intelligence, appending the new information on the graph.
BlackSwan’s knowledge graphs enable boundless flexibility to append new attributes to existing entity data points via nodes and vertices, as well as to append new entities or modify existing entity nodes and attributes. The enrichment functionality of our knowledge graphs also automatically fills in missing information via an advanced search of internal and external sources.
- Automating construction via a Data Fabric
BlackSwan’s Knowledge Mesh provides the scaffolding to automate the construction of knowledge graphs for any business application by providing the domain context.
Unlike traditional knowledge graphs which are built using extract, transform, and load (ETL) pipelines; our knowledge graphs access data through a Knowledge Mesh architecture — our advanced implementation of the Data Fabric concept.
The issue with an ETL pipeline is that it is based on traditional data integration platforms which take a centralised approach, and this leads to some big challenges – namely data silos, duplication of work and overreliance on technical subject matter experts.
In contrast, Knowledge Mesh takes a decentralised, domain-oriented, metadata-focused approach. It incorporates data enrichment and data virtualisation, so that data assets can be pulled from any operational source, with no need to replicate.
Crucially, the Knowledge Mesh utilises a product catalog, allowing swift maintenance of best practice values and data models of business domains. All this circumvents the cumbersome need to build knowledge graphs from scratch.
BlackSwan’s ELEMENT™ for innovative knowledge graph applications
BlackSwan’s flagship platform ELEMENT™ utilises our proprietary knowledge graph technology to manifest networks of structured and unstructured data from any internal or external source.
BlackSwan recently won an award for Most Innovative Knowledge Graph Implementation in the A-Team Innovation Awards 2022. Our Knowledge Graphs are schemaless, enhanced with Composite AI capabilities and come equipped with an Insight Engine and continuous updates. Businesses can use Knowledge Mesh to automate the construction of knowledge graphs, enabling them to create any business application with the required domain context, with ease.
Learn more about our Knowledge Graphs, in this article on IDG Connect.
Learn more about BlackSwan Technologies’ ELEMENT™ here.
Nadee Wije is a Copywriter at BlackSwan Technologies. Certified in copywriting and content marketing, she has over 3 years of experience writing sales and marketing content for emerging B2B technology companies across the globe.
Follow BlackSwan on Twitter and LinkedIn