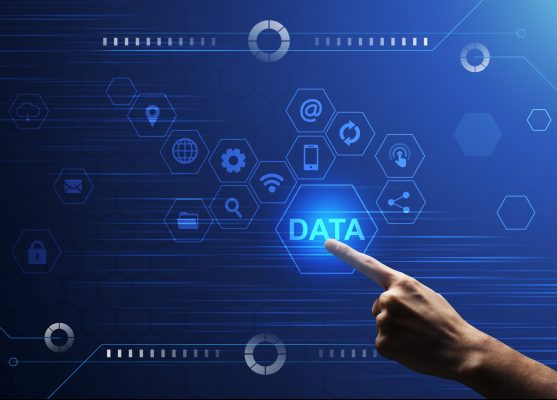
May. 12, 2021
Why the Gap Between Enterprise AI Deployment Ambitions and Reality?
By Scott Lichtman @BlackSwan Technologies
Several surveys (including two published in April 2021) about the state of enterprise-wide artificial intelligence shed light on where corporations are succeeding with AI, yet how they are struggling to deploy the technology pervasively.
This overview summarizes findings from Deloitte, O’Reilly and Juniper, based on their surveys of thousands of business and IT leaders across the globe. The most valuable, common insights have been condensed here, followed by recommendations for making greater headway with AI in your organization.
AI Projects Accelerate but Widespread Deployment in Enterprises is Elusive
Juniper Network’s survey concisely addresses the fundamental paradox. While 95% of business leaders recognize the value of deploying AI across their organization, only 6% of them felt they had achieved ‘widespread’ enterprise adoption.
Why the gap between AI deployment ambitions and reality? One might attribute it simply to the still-early maturity of the AI sector; that broader adoption will come easily once pilot projects have delivered demonstrable savings in several departments and Operations & IT leaders have multiple deployments under their belts. However, there are specific challenges that can be proactively addressed by enterprises ready to expand their use of AI.
Regarding technology, the top challenges to broader adoption were:
- Data quality and building a flexible data platform that supports the voluminous, complex data needs of AI models. Juniper found that, after AI technologies themselves, the most important enabler of AI was high-quality data availability.
- Achieving transparency and process replicability, cited by both Deloitte and O’Reilly. AI project management disciplines are still being devised ad hoc at the departmental level, while AI models themselves haven’t made explanation, documentation, or extension easy.
- Integrating existing technologies and workflows with the AI tools. Too often AI products act like closed systems, at best with minimal exchange of information through limited APIs.
Process-wise, companies had difficulty:
- Identifying use cases with high ROI. 49% still find this to be a problem, according to O’Reilly. This reflects not only the novelty of the cognitive computing & ML paradigms, but also the difficulty of predicting adoption.
- Transforming a pilot into a well-integrated and reliable production solution. AIOps, the discipline of proactively coordinating AI model development with efficient deployment and maintenance, is still in its early stages. Many cloud deployment steps are based on lengthy scripting and skill-intensive model and data management.
Top people-related challenges are:
- Investing in hiring and (re-)training employees versed in applying AI. O’Reilly found that 52% of companies were unable to recruit enough data scientists and ML modelers. Juniper noted that 41% of respondees had made it a top priority this year to bring up-to-speed both technical and non-technical – business analyst and end-user – staff on AI.
- Establishing corporate guidelines around the ethical use of AI and access to the results. In Juniper’s findings, 89% strongly agreed that firm governance and ethical considerations were necessary for regulatory, reputational and societal reasons. However, only the most mature organizations, 20% of respondees, mainly those with commercial deployment, were making governance a top priority for 2021.
A (Word) Cloud of AI Adoption Considerations
Related technology trends involve the Cloud, Cybersecurity and AI-Embedded Apps
In addition to the top challenges above, several detailed findings are noteworthy.
- The cloud is being leveraged heavily for running applications and processing data, due to its ease of deployment and high degree of scalability. Deloitte respondees ranked cloud-use third in a list of tactics to accelerate deployment (after AI tool acquisition and data framework development).
- Related to the cloud are greater concerns about cybersecurity – hacking into data sets or altering models. In Juniper’s survey, cloud capabilities and robust cybersecurity were the third and fourth most important technology capabilities, after AI tools and data management. Customer data privacy and permitted use were noted as related concerns.
- Increasingly, experienced adopters are buying ready Ai-embedded platforms and applications more than building their own solutions using heterogeneous tool sets. The percent in Deloitte’s study that prefer to buy instead of build has crept over 50% over the last 3 rounds of surveys, as cloud-based, commercial platforms now incorporate more of the many activities needed to extract business value from AI.
Reflection and Inflection in 2021 – Deployment Recommendations
2021 could be the turning point in the global shift towards broad deployment, as O’Reilly’s top response to “AI Goals for 2021” were “developing AI models and data sets that can be used across the organization.” Deloitte more provocatively opines that the era of early mover advantage with AI is ending soon, as organizations learn to systematize opportunity identification and solution delivery.
61% of leaders surveyed by Deloitte felt that their entire industry would be transformed within three years by AI.
We suggest the following strategies to become, or remain, an innovation leader.
- Apply a Data Mesh framework. A Data Mesh architecture acknowledges that data is best captured and maintained from their original source and user base… as opposed to compiling a monolithic data warehouse or data lake. The mesh applies metadata and uses machine learning to build a dynamic, distributed overarching layer to coordinate those data resources. It can equally handle structured data, text, edge-based data streams, and multi-media. This architecture serves as the basis for well-maintained, accessible data, which is among the very top challenges to enterprise-wide adoption.
- Combine cognitive computing with other AI technologies for greater business staff involvement and model transparency. Cognitive computing refers to symbolic AI techniques that assist people with decision-making and are generally interpretable directly by those people. A knowledge graph is a good example; it uses a network representation to depict industry terminology; organizations, people and other entities; characteristics about them; relationships they’ve formed; and activities they have performed. They are developed through a combination of a universal (i.e., business/economic conceptual) framework, customised specifications by an organisation’s business analysts; and automation to extract domain meaning from related content. Most importantly, knowledge graphs and their application to decision-support are fully transparent. They can be browsed through visual link analysis, refined with expert input specific to a user role or business department’s needs, and decisions based on calculations made on the graph are explainable. This serves as a complement to machine learning, in which the inner workings of a model are harder to explain and are dependent on ML engineers and big data scientists to develop.
- Prototype quickly and succeed (or fail) fast. It’s difficult to predict the value of an AI project without a sense of what insights can be extracted from the data. Tools are now available that allow for the application of AI models pre-purposed for common business needs like anomaly identification or customer segmentation. Combined with connectors that provide ready access to many data sources, the pre-built models can ingest data and deliver live AI findings almost immediately. After this Proof of Concept, a full pilot should be achievable in several weeks. To plant seeds, identify new opportunities and achieve ROI quickly, focus on agile concept-to-launch methodologies, rather than grand designs for digital transformation that can take years to realise results.
- Assess solutions for Native Cloud Engineering. An increasing number of AI tools take advantage of cloud deployment for data processing scale. To leverage the cloud to the utmost, the AI offering should be deployable across multiple cloud platforms for future flexibility; leverage cloud services including API gateways and security/authentication best practices; and support ALOps/MLOps for seamless deployment of models.
- Look for solutions that combine leading AI technologies with enterprise business functionality. This includes workflows, alerts, customer/case management, data visualisation dashboards, customer self-service, and collaboration features. The market is witnessing a new approach to enterprise business software, in which the emphasis is on greater, more pervasive intelligence support for team decision-makers. The previously rigid structures that dictated which fields, screens or processes are presented by the system are giving way to more fluid problem-solving and collaboration built around explorable insights.
- Appoint a corporate AI Officer – the lead, responsible party to coordinate corporate AI strategy, including “moonshot” project selection, budget, risk identification and ethics consideration, and usage / access governance. The same person should evangelize the most effective tools and processes to engage all the business lines and IT and find synergies. In Juniper’s study, 7% of respondees have taken this step so far with more under consideration.
An AI-driven future for businesses is no longer “just around the corner;” it’s now “knocking at your door.” While there’s opportunity ahead to become a truly AI-driven enterprise, the time has arrived to develop one’s coordinated strategic plan for deploying AI broadly, flexibly and ethically.
Learn more about how BlackSwan Technologies’ ELEMENT™, the world’s first AI Operating System for business, supports the key requirements for systematic, enterprise-wide adoption of artificial intelligence.
Scott Lichtman is a Marketing Director at BlackSwan Technologies. He has worked in strategy at Oracle and has helped lead numerous startups. Scott holds a computer science degree from MIT, an MBA from Harvard and a Masters in International Economics from LSE.
For similar content, follow us on Twitter and LinkedIn